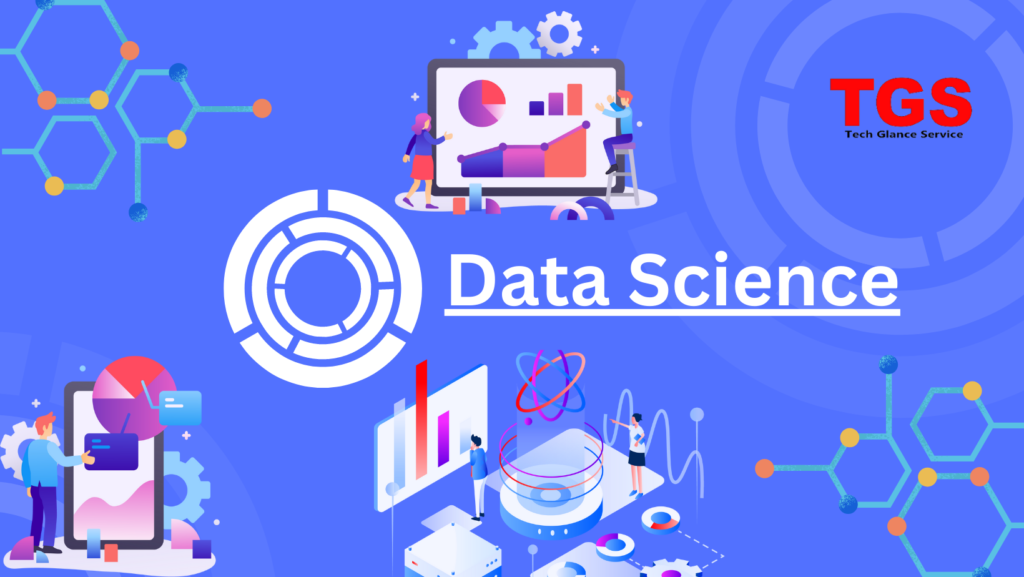
What is Data Science?
Data science is an interdisciplinary field that involves extracting insights and knowledge from data by using a variety of statistical, computational, and analytical techniques. It combines elements from various fields such as mathematics, statistics, computer science, and domain-specific knowledge to find patterns and trends in data.
Data scientists use a range of tools and techniques to collect, process, and analyze large volumes of data. They may work on various stages of the data analysis process, including data cleaning, data preparation, exploratory data analysis, modeling, and evaluation.
Data science is used in a wide variety of fields, including business, healthcare, finance, marketing, and more. Some common applications of data science include fraud detection, recommendation systems, predictive analytics, and machine learning.
What does a Data Scientist exactly do?
Data scientists are responsible for extracting insights and knowledge from data by using various analytical techniques. Here are some of the key tasks that a data scientist may be responsible for:
- Data collection and cleaning: Data scientists work with large volumes of data, which may come from various sources. They need to collect and clean this data to ensure that it is accurate, complete, and consistent.
- Data exploration: Data scientists use techniques such as data visualization and statistical analysis to understand the data and identify patterns and trends.
- Modeling and analysis: Data scientists use various modeling techniques, such as regression analysis, clustering, and machine learning, to build models that can be used to make predictions or identify insights.
- Communication and collaboration: Data scientists need to communicate their findings to stakeholders, including executives, business analysts, and other team members. They may also collaborate with other data scientists, engineers, and analysts to develop solutions and improve data infrastructure.
Overall, data scientists play a critical role in helping organizations make data-driven decisions and develop new insights that can drive innovation and growth.
What is Data Science used for?
Data science is used to extract insights and knowledge from large volumes of data to help organizations make data-driven decisions. Here are some of the common applications of data science:
- Business intelligence: Data science is used in business intelligence to analyze large volumes of data and provide insights that can inform business decisions. This may include market research, customer segmentation, and sales forecasting.
- Machine learning and AI: Data science is used in machine learning and artificial intelligence (AI) to train models that can automate tasks, identify patterns, and make predictions.
- Healthcare: Data science is used in healthcare to analyze patient data and develop new treatments and therapies. It may also be used to monitor public health trends and develop policies to address health concerns.
- Finance: Data science is used in finance to detect fraud, identify market trends, and manage risk. It may also be used to develop new financial products and services.
- Marketing: Data science is used in marketing to analyze customer behavior and develop targeted campaigns. It may also be used to optimize pricing and promotions.
- Natural language processing: Data science is used in natural language processing to analyze and interpret human language. This may include sentiment analysis, chatbots, and speech recognition.
Overall, data science is used in a wide variety of industries and applications to extract insights from data and make better decisions.
What are the benefits of Data Science for business?
Data science can bring several benefits to businesses. Here are some of the key advantages:
- Improved decision-making: By using data to inform decisions, businesses can make more informed and accurate decisions. Data science can help identify patterns, trends, and insights that might not be apparent through other methods, allowing businesses to make more effective decisions.
- Increased efficiency: Data science can help automate repetitive tasks, reducing the need for manual labor. This can lead to increased efficiency and productivity, allowing businesses to accomplish more in less time.
- Competitive advantage: Data science can help businesses gain a competitive advantage by identifying opportunities and trends that their competitors might miss. By using data to make informed decisions, businesses can stay ahead of the curve and innovate more effectively.
- Better customer understanding: Data science can help businesses better understand their customers by analyzing their behavior and preferences. This can lead to more targeted marketing campaigns and a better overall customer experience.
- Improved risk management: Data science can help businesses identify and manage risks more effectively. This can include identifying fraudulent activity, predicting equipment failures, or managing supply chain disruptions.
Overall, data science can provide businesses with a wealth of insights and advantages that can help them stay competitive and innovative in today’s rapidly changing business environment.
What is the Data Science process?
The data science process is a systematic approach to extracting insights and knowledge from data. The process can vary depending on the specific use case and the organization’s needs, but here are the common steps that make up the data science process:
- Problem formulation: In this first step, the data scientist works with stakeholders to identify the problem they want to solve. This step includes defining the problem statement, identifying the key business questions, and determining the data sources that will be used.
- Data collection: The next step is to collect the data that will be used to solve the problem. This may involve collecting data from internal sources, external sources, or generating new data.
- Data preparation: Once the data is collected, it needs to be cleaned, formatted, and prepared for analysis. This step includes removing missing values, handling outliers, and transforming the data into a format that can be analyzed.
- Data exploration: In this step, the data scientist explores the data to understand its characteristics and identify patterns and trends. This may involve data visualization, descriptive statistics, and data mining techniques.
- Modeling: After the data is prepared and explored, the data scientist selects the appropriate modeling technique to build a predictive or descriptive model. This step involves selecting the appropriate algorithm, parameter tuning, and model validation.
- Evaluation: In this step, the data scientist evaluates the performance of the model to determine its effectiveness. This may involve measuring accuracy, precision, recall, and other metrics.
- Deployment: The final step is to deploy the model into the production environment, where it can be used to make predictions or provide insights to stakeholders.
Overall, the data science process is a cyclical process that involves iteratively refining the problem formulation, data collection, and analysis until the desired outcome is achieved.